AI in healthcare, separating facts from fiction
on LinkedIn:
James Baker, partner at Cambridge Design Partnership, considers the future for AI in the real world with help from a sideways look at its portrayal on the big screen.
In the movies, we often see big tech and deep data combine to challenge humankind in new and ever more fiendish ways. Indeed, at the cinema, human interaction with Artificial Intelligence (AI) is a rich seam of storytelling, which rarely ends well, for the human!
Meanwhile, back in the real world, we are now in an era where digital data, and more importantly the insights that can be drawn from it, can be as important – and as valuable – as physical objects. At Cambridge Design Partnership (CDP), one of our specialisms is the design of medical devices, often using information and machine learning to provide utility and value beyond the physical device alone.
So, in the spirit of fun, here is what the silver screen tells us about the big questions surrounding machine learning in healthcare, and we ask how these ideas relate to the reality of what the technology can achieve today?
What price genetic data? (Gattaca)
In the 1997 film Gattaca, only genetically perfect humans are eligible for better jobs and lifestyles. We cheer on Ethan Hawke’s ‘genetically inferior’ character as he assumes the identity of a superior being in order to become an astronaut.
In today’s world, less than 20 years since Gattaca was filmed, genetic profiling and statistical prediction is gathering speed. Mapping of genomic sequences to traits is a rich area of study and just this week, Matt Hancock the UK Health secretary announced that all babies could receive a complete genome sequencing at birth. Crucially, this technology has the potential to predict an individual’s likelihood to suffer illness in the future. But should the way you are treated as a patient, or indeed a person, be determined by an assessment of your genetic makeup? Already insurers are asking for access to medical records and premiums are affected by the presence of certain diseases, so should they also be able to consider the likelihood of future illness as well?
Diagnosis – how far should you go? (Minority Report)
The film Minority Report envisages a world in which arrest and incarceration is based on a prediction of the likelihood to commit a crime before it has occurred.
Already today’s healthcare and wellness technologies create significant amounts of data about individuals. New processing methods and machine learning can analyse these multiple sources and draw conclusions.
Yet many clinicians don’t want every possible analysis to be given to them. For example, who is responsible if systems predict the probability of an illness, but the medical practitioner can’t confirm this conclusively? Does informing the patient provide any utility?
There are recent moves to define what can and can’t be done with personal data, such as the European Union’s General Data Protection Regulation (GDPR). These seek to control access to and ownership of data, but as yet, there are no similar frameworks to control the conclusions drawn from it.
What if AI overtakes human intelligence? (Ex Machina)
In the film Ex Machina a humanoid robot is created and given ‘intelligence’ built using a record of billions of human internet searches. But then (surprise!) the robot uses its knowledge of human interactions and desires to achieve its own freedom, deliberately misleading its human masters to do so.
Machine learning using huge amounts of information is an approach we see increasingly used in real life. In the field of diagnostics, AI is already showing great promise in diagnosing conditions such as Alzheimer’s and in facilitating cancer diagnoses. AI predictions are compared with a gold standard diagnostic to determine the most significant automated metrics to detect the condition.
This approach is already being used in cancer screening, enabling earlier detection through far more extensive analysis than is possible manually.
But what if AI doesn’t react like we expect? (2001)
An all time classic, 2001 cleverly hides a story of unintended consequences within a ground breaking and spectacular space opera. The HAL character appears to have a sinister agenda and behaves malevolently, attempting to kill off the human crew – but ultimately is understood to have been driven by conflicting orders.
In the real world, AI can deliver responses that are not what we expect. Large data sets may still contain insufficient information, erroneous or poor-quality data, which by chance may create patterns that have no meaning.
A good example of where AI can deliver unanticipated (and unwanted) behaviour is the late, unlamented Microsoft Tay chatbot. Its premise was that, by listening to and learning from posts on Twitter, it could generate useful tweets and help manage commercial Twitter accounts. But within hours of its release in 2016, Tay began posting inflammatory and offensive tweets and had to be taken down.
So, before we make AI systems independent, how can we be sure how they will behave and who takes responsibility for their actions?
Sometimes, AI can really help us (Wall-E)
The 2008 story of a good-natured planetary janitor-bot left to clean up our human mess shows how AI can really benefit humankind, turning its hand to automating work that would otherwise be onerous and low value. See also, C-3PO and R2-D2 in the Star Wars movies. It’s surely no coincidence that the two loveable droids are the only characters to appear in every single film in the Star Wars franchise.
Back in 1950, computing pioneer Alan Turing predicted that by the year 2000 computers would be able to trick us into believing they were human 30% of the time. He was not far wrong, in 2014 a chatbot called Eugene Goostman convinced 33% of judges that “he” was a 13-year-old from Ukraine, thus officially passing the Turing Test. We see these kinds of natural language interaction technologies being used increasingly in consumer goods, but also finding utility in medical applications such as triage with patients seeking care. This enables faster access and a better “customer experience” whilst also allowing healthcare practitioners to focus on provision.
In conclusion, at CDP our focus is on how to realise value for our clients, and machine learning is one of the tools we can bring to bear. With the ongoing bombardment of new technologies, it is important to understand when it can provide effective solution, and when more traditional methods will provide the best results. It’s no longer a question of what can we do with AI?
We need to ask: What should we do?
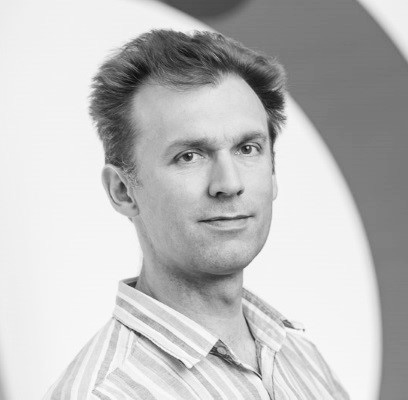
James Baker
Partner & Electronics Engineer