COVID-19 Modelling
Models are everywhere – anyone who has played a computer game has encountered a model, but in a pandemic, mathematical models are vital for understanding the dynamics of transmission, disease progression, healthcare needs and the overall outcome on the population.
Recent real world events have shown that critical decisions are being taken based on model data. Many people are still uncertain of how models work which can lead to them being met either with undue suspicion or absolute faith. In reality models are incredibly powerful, as you can test different courses of action quickly so an optimised response can be formulated, but their limitations need to be understood.
What is modelling?
Here we try and explain the basic principles of models, how mathematical models can help and what their limitations are.
Mathematical modelling involves making a mathematical representation of a system where the expected outcome of changing various parameters can be calculated. This means you can examine the outcomes of many scenarios using the same model. For example, the models reported on recently look at the spread of Covid-19 with different levels of social distancing and other interventions such as school closures. A model of a complex system is usually a collection of many separate models, each a breakdown of a different part of the system. For example, the first thing to consider when modelling the spread of disease is infection. You need to know certain things such as;
- how likely is the infection to spread person to person with each contact?
- how often do people come into contact with each other?
These values are called parameters and can be changed depending on the situation. The equations behind epidemiological models for the spread of infection are well established, but the parameters will vary between countries depending on things like the breakdown of age and population density. Crucially, epidemiological models rely on data. Sourcing these parameters is all part of modelling and assumptions have to be made.
For recent UK modelling this data came from a number of diverse sources. Census data could give a good indication of the age and distribution of households, data on social connections broken down by type (work, school, home) and age came from a BBC citizen science project. Even knowing this, data on class sizes, commute distance and company size were then needed to create a virtual population to simulate the spread of the disease.
Models can also show where collected data may be inaccurate. Recent models monitoring the situation in Spain found that the number of COVID-19 deaths reported appears to be significantly underestimated, given existing data on the expected seasonal number of deaths in a normal year and data on the total number of deaths recorded in the past few weeks (by any cause).
This is a very simple model purely looking at infection. When ‘infected’ balls come into contact with others susceptible to the disease there is a probability that the other balls will become infected. There is no death rate, everybody recovers and is then immune. The speed of the balls represents the number of social contacts. The probability of infection, the proximity for infection, the length of the infection all need to be set. Even in this toy model many assumptions have been made.
What changed with the recent modelling?
Having modelled infection and the population, the outcome needs to be considered. What proportion will recover and develop immunity? What proportion of people will become hospitalised? Of them, crucially, how many will need intensive care and specialist equipment like ventilators. All these parameters depend on the disease itself. Unfortunately, Covid-19 is practically unknown, researchers have had only a few months to study it. That means there has been a degree of uncertainty with the parameters fed into the models.
One widely reported model that looked at the impact on the UK population was led by Imperial College London. Having updated their models with better information from Italy on the proportion of patients requiring intensive care beds, it found that the UK’s National Health Service would not be able to cope without further action, which led to the recent dramatic change in government policy.
In the report, the team presents a pandemic curve for different degrees of potential government intervention. The different measures that they considered the impacts of were: no intervention, household isolation, social distancing, and school closures. With no intervention, the model predicted a need for hospitalisation thirty times what the current UK healthcare system can manage. Only by combining all of the measures would the healthcare system not be overwhelmed.
This is one of the graphs from the Imperial paper [1]. The vertical axis shows the number of critical care beds needed through time in each scenario. The blue region shows the time period on the horizontal axis where various social distancing is applied. The horizontal red lines show the maximum number of NHS critical care beds available. The various lines, explained in the key, show the difference between doing nothing, applying some social distancing and with full school and university closures. This graph shows that without all measures being taken the number of available beds would be exceeded.
What happens next? What about the large peak when the measures are withdrawn?
We need time. Time to get better testing, time to find new treatments and more ventilators. The current model suggests the current restrictions should keep the number of cases at a manageable level for the next few months.
The Imperial model makes important assumptions. Firstly, it assumes that measures put in place to control the spread of the virus are all lifted at the same time, which is neither realistic nor advisable. Secondly, it assumes that recurrences of the outbreak after the initial lift of restrictions continue for an indefinite period. In reality, this may not happen due to people acquiring immunity or the availability of a vaccine. Finally, it doesn’t account for infected cases that have gone undetected or for tools such as contact tracing, which can help break the chain of transmission. All of this will affect the number of people who become infected once the measures are lifted.
A competing model by Oxford University followed the publication of Imperial’s model. This model stated that under-detection of cases could be high, indicating that a significant part of the UK population could have already contracted the virus. This caused a big media response, however, given the data available it seems an unexpected conclusion to draw, as epidemiologist Adam Kucharski pointed out.
The Imperial model has since been refined and other competing models have been published, but the consensus remains.
It’s important to remember that the model is only based on what we know now. Models are continuously updated. Research teams are now focusing on measuring the impact and preparedness of healthcare systems by predicting the number of hospital beds, ventilators and testing kits needed based on what the models are telling us. They are also looking at the big question of the length of time needed before lifting restrictions and how to prevent a second wave of the outbreak, and models will help us to understand this better.
So in a few months the situation will have changed, the model will be updated with more information and the curve may look very different. Data should start to emerge to confirm level of immunity gained from recovering from the disease. More hospitals are currently being built and there is a national effort to produce more ventilators. Doctors have already identified the response that causes some patients to develop severe symptoms while most have a mild version, which may make it possible to screen people to detect who is most vulnerable or identify better treatments.
Given the rate of learning in the last 3 months the picture may be very different when more accurate assumptions are fed into the models.
Once a vaccine has been developed, we may require modelling for rolling it out to best effect, as well as monitoring changes in the virus. This will feed into the ongoing body of research for this pandemic and will help us prepare for future pandemics.
While models are not always accurate, they help build a consensus of understanding that informs policy and helps save lives. Taking the right measures at the right time is key in the fight against Covid-19 and through modelling we can make the best-informed decisions possible from the data available.
References
[1] https://www.imperial.ac.uk/media/imperial-college/medicine/sph/ide/gida-fellowships/Imperial-College-COVID19-NPI-modelling-16-03-2020.pdf
[2] https://www.gov.uk/government/groups/scientific-advisory-group-for-emergencies-sage-coronavirus-covid-19-response
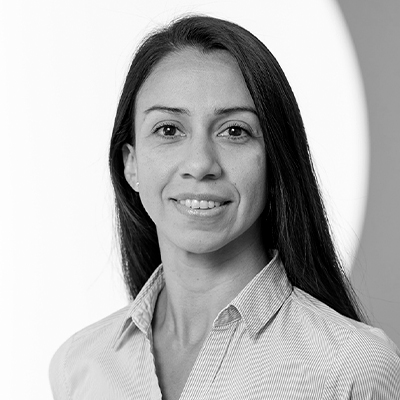
Karla Sanchez
Biomedical Engineering Consultant
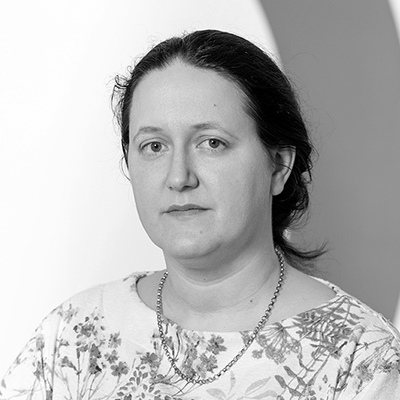
Alison Hart
Consultant Data Scientist
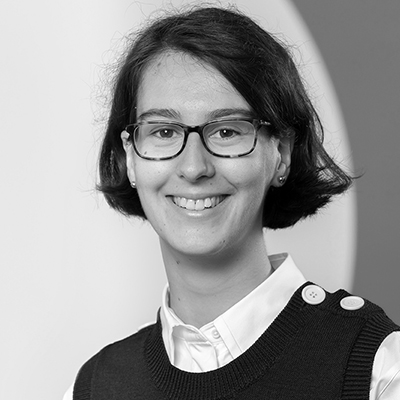
María Fernández-Martos Balson
Biomedical Engineer